THANK YOU FOR SUBSCRIBING
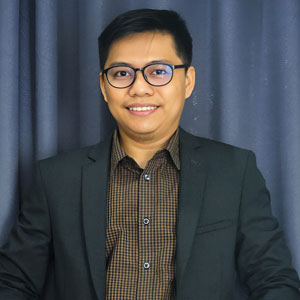
Agus Jamaludin, Data Scientist Lead, Petrosea
Agus Jamaludin is a seasoned Data Scientist with over nine years of experience, specializing in data architecture, pipeline development, predictive analytics (ML/AI) and data visualization. As the Data Scientist Lead at Petrosea, he drives datadriven decision-making by designing scalable data solutions, optimizing operations with predictive models and developing interactive dashboards using Power BI and SAP Analytics Cloud. Agus collaborates closely with IT, operations and business units to align data strategies with business objectives.
Through this article, Jamaludin highlights the need for a blend of technical, business and leadership skills to drive innovation and efficiency in engineering data science.
Key Skills for Data Scientists in Engineering
In the engineering sector, a data scientist must combine technical and business skills to drive operational efficiency, predictive maintenance and data-driven decision-making. Data engineering and pipeline development are essential for handling large-scale sensor, IoT and operational data while selecting the correct big data or traditional technologies for efficient processing. Advanced analytics and modeling are critical in defining the right analytical approach based on business goals and data characteristics, whether structured, unstructured, time-series, or geospatial. Data visualization and BI tools are crucial for effectively communicating insights through dashboards and reports, ensuring that analytics drive informed decision-making. Equally important is business and domain knowledge, particularly in mining and engineering operations, to apply analytics in key areas such as equipment reliability, safety, production planning and resource optimization. A strong understanding of cost optimization, risk management, stakeholder communication and compliance ensures that AI-driven solutions align with business objectives while adhering to industry standards.
Challenges in Engineering Data Science
From a technical perspective, data science challenges in engineering services are similar to those in other industries. These include handling noisy data that requires extensive cleaning and managing massive datasets efficiently to optimize resource allocation and costs. From a business perspective, engineering services present unique challenges. Heavy machinery failures can cause significant downtime and financial losses, making traditional preventive maintenance inefficient. By integrating engineers' expertise with analytical approaches like anomaly detection and machine learning, we developed predictive models to estimate the Remaining Useful Life (RUL), optimizing maintenance schedules and reducing costs.
Resource allocation is another critical challenge in engineering projects. Poor equipment, labor and materials optimization leads to cost overruns and delays. We addressed this by developing dynamic optimization models for resource allocation, such as trucks and excavators.
Additionally, many engineering professionals are unfamiliar with AI/ML, leading to resistance to adopting data-driven insights. To build trust, we collaborated closely with engineers, integrated models into user-friendly dashboards and conducted training sessions to facilitate adoption.
Leadership for Innovation and AdaptabilityAt my company, we embrace the ACTION core values shaping my leadership approach. "A" for Agile means fostering flexibility, continuous learning and quick adaptation to industry changes. "I" for Innovative encourages creativity, experimentation and leveraging advanced data science techniques for impactful solutions. "O" for Open-Minded promotes knowledge sharing, cross-functional collaboration and openness to diverse perspectives. By embedding these principles, I ensure my team remains innovative, adaptable and resilient in a fast-evolving industry.
To excel in data science, focus on strong fundamentals in mathematics, statistics and machine learning—understanding the "why" behind models is key. Business and domain knowledge are just as crucial; knowing engineering, mining, or manufacturing workflows sets you apart
In my experience, several advanced analytics and AI techniques have been highly effective in optimizing engineering processes, particularly in mining, construction and heavy equipment management. For instance, predictive maintenance, which combines the knowledge from the engineer with machine learning using IOT sensor data, helps us anticipate failures before they happen. This reduces unexpected downtime, maintenance costs and operational disruptions. AI-Driven Resource Allocation, which AI optimizes resource allocation, ensures equipment trucks and excavators are used effectively to reduce bottlenecks and enhance productivity.
The Role of Automation in Data ScienceAutomation is crucial in streamlining data science workflows, ensuring efficiency, scalability and repeatability. Given the complexity of engineering and industrial analytics, automation helps reduce manual effort, minimize errors and accelerate insights. Automating ETL (Extract, Transform, Load) processes ensures that data is ingested, cleaned and transformed in a structured manner. This reduces data inconsistencies and allows for real-time analytics. Automating feature engineering speeds up model development by identifying the most relevant variables. Automated hyper-parameter tuning ensures optimal model performance without manual intervention. MLOps automates model deployment, versioning and monitoring, ensuring that models remain reliable and up-to-date.
Advice for Aspiring Data ScientistsTo excel in data science, focus on strong fundamentals in mathematics, statistics and machine learning— understanding the "why" behind models is key. Business and domain knowledge are just as crucial; knowing engineering, mining or manufacturing workflows sets you apart. Storytelling and visualization skills help translate complex insights into actionable decisions for stakeholders. Automation and MLOps are essential for scaling AI solutions, so mastering CI/CD, data pipelines and containerization ensures production readiness. Stay resilient—real-world data is messy and failure is part of the process. Keep experimenting, learning from setbacks and refining your approach. Lastly, network and stay updated through conferences, hackathons and online communities. The field evolves rapidly—adapt, innovate and enjoy solving real-world problems with data!
Weekly Brief
I agree We use cookies on this website to enhance your user experience. By clicking any link on this page you are giving your consent for us to set cookies. More info
Read Also
Ethics & Compliance in a Digital World: Navigating HCP Engagement in APAC
How AI is Driving Innovation and Customer-Centricity in Insurance
The Impact of Smart Infrastructure on Growing Cities
Transforming Engineering through Data Science
Redefining Risk Management: Strategies for a Safer Future
New Hr Capabilities To Face Evolving Technologies
Strengthening The Compliance Fortress In The Banking Sector
Navigating Legal Challenges By Adapting To Technological Shifts
